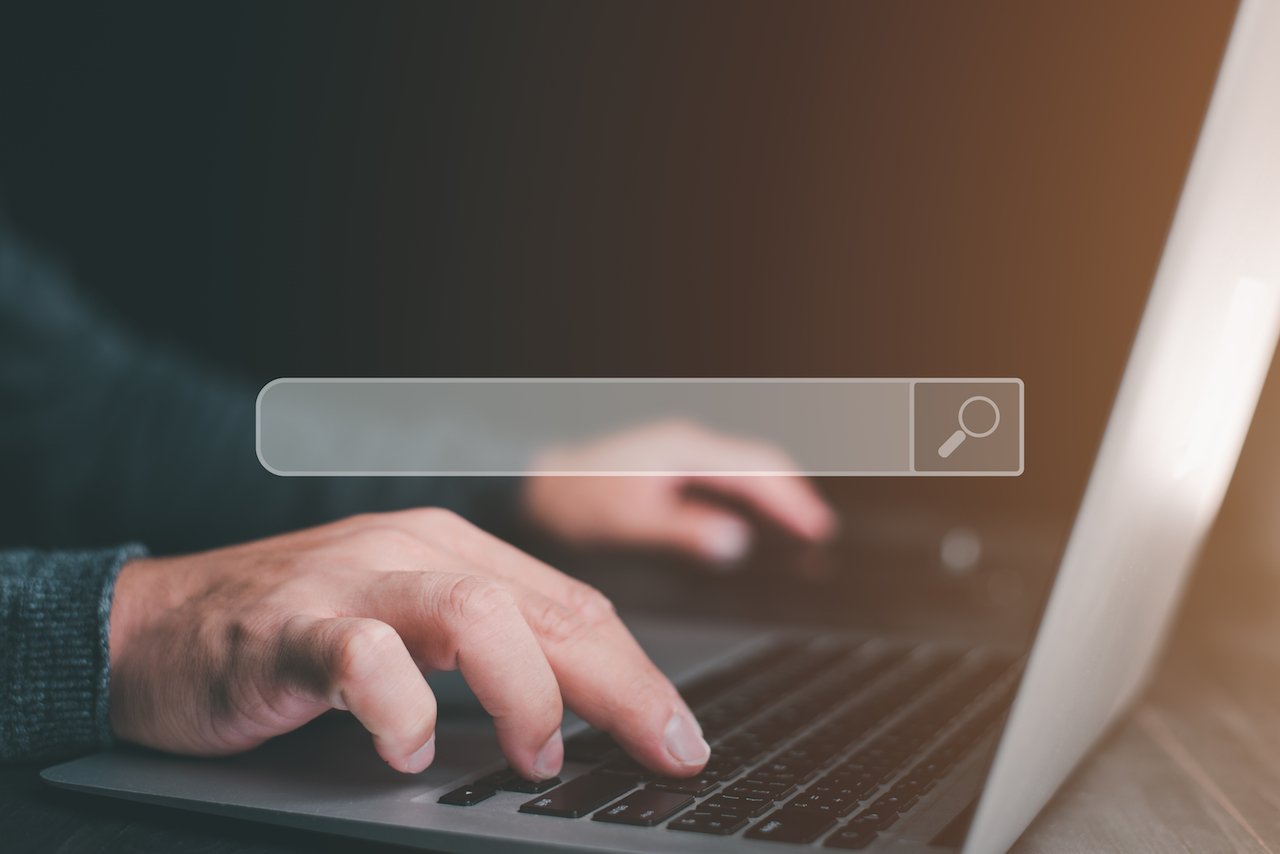
How Generative AI Can Improve Enterprise Search
I was inspired to write this post after listening to an episode of “This Week in Windows” on Leo Laporte’s TWIT.TV podcast network. Leo and one of his co-hosts got into an interesting discussion on the use of Generative AI like ChatGTP with respect to search on the internet.
Leo seemed to be making the same mistake many do, and confusing the concepts of using a search engine to answer a query by finding sources of information, and asking a Generative AI system based on a Large Language Model (LLM) to answer a question. The two things are similar but not the same, and what was being discussed was actually adding ChatGTP to Bing, bringing two technologies together to search for information.
Search Engine versus ChatGPT
How are the two things different, and how are they similar?
Well, first let's simplify things by considering the vast corpus of data used to train an LLM and the potentially equally vast index of web search engines as “databases” that are being queried when you ask the systems a question.
A major difference is that GPT 3.5 was trained on information published up to 2021. While it’s training data may have included sources on the internet, asking ChatGTP a question that about something that happened last month is not going to work, because that information is not in its ‘database’. Using an up-to-date search engine that is periodically crawling all its sources to update its index is going to bring back the latest information, because it is in its ‘database’.
Language: The Strength of ChatGPT
However the strength of ChatGPT and an LLM is in the middle L – Language.
One of the reasons for developing LLM’s was to understand and process natural language. So rather than typing a few keywords into a Google or Bing search box like most of use have been conditioned to do, an LLM system like ChatGTP is used to provide a “conversational interface” to a search engine.
The user can be much more “loosey goosey” with their query, and of course they can “chat” with the system via this user interface, expanding on their question, or asking follow up questions. Using Bing as an example, the natural language responses to the users’ questions have the web sources included in them as references, which can be clicked on to directly visit the source of information.
Impact of Generative AI in Enterprise Search
So, what does this have to do with enterprise search? While listening to, and trying to understand Leo’s confusion, I got to thinking about some recent conversations about the impact of Generative AI on Enterprise Search, specifically, and how it differs from other AI tools which have been used to enhance search tools for years.
Organizations have used tools like Entity Recognition and Machine Learning models for content classification for years and, as a result, inspired industry analyst reports like Forrester’s WAVE is for ‘Cognitive Search’ and Gartner’s Magic Quadrant is for ‘Insight Engines’.
Generative AI capabilities based on LLM’s offer the same advantage in the enterprise context as they do for web search. A couple of weeks ago I was lucky enough to be at networking group meeting hosted by the digital workplace team at a large Canadian bank. They have just released a ChatGTP based search capability internally, that provides the same advantages of a conversational UI as for web search. It includes a relatively ‘huge’ query box, with a lot of text in it explaining to users that the more they write, and the more specific they can be, the more it will help in finding good results – a great example of a helpful user experience, a form of prompt engineering I suppose. Some of us geeks actually use the ‘advanced search’ on various systems, including the intranet, and we might select various options from drop downs or type choices into boxes; like selecting Word documents and PDF’s as the information format, maybe a document type, or a department, in order to narrow down our search. We may or also be more, or less skilled at using facets or filters based on metadata to narrow down the results that are presented to us (if the system provides these features of course). However, for those who are not information search and retrieval geeks, the ability to lever that conversational interface to use natural language to ask things like “show me the quarterly sales report for the blue widgets for the eastern US region from may 2020 until now” and to narrow down the presented results by asking follow up questions is a great leap in user experience; but if conversational UI’s are the front end of enterprise search, how can Generative AI help on the back end.
If you know me, or have ever read anything I have written, you will know I am a big metadata nerd, and remain convinced of the absolute value of metadata to enterprise search – the more the merrier! Now we have been able to use Discriminative AI, in the form of Machine Learning models to categorize content for a long time, and it is a valuable tool. People do not like having to fill in metadata fields manually, so my admonition of the more the merrier just doesn’t wash with the average end user! So the more automatically generated metadata the better, and LLM based Generative AI can help here too. While ‘old skool” ML systems need to be trained to categorize certain content or documents, and append that categorization metadata to the index, LLM’s can do a lot more, again because they are designed to understand and work with language. LLM’s are great for pattern matching, but at huge scale, so not only can you use them as part of your indexing pipeline but you can use them to scan all your existing intranet pages, documents and other systems, adding metadata to your index, including good quality summaries. So, to add a final point, I will just note that summary metadata is getting more and more important as organizations decide to encrypt more documents and information for security, privacy and other regulatory reasons. Some systems, such as the DMS provided by my old employer NetDocuments do very clever things so that you can search across encrypted documents, but on the other hand M365 customers are finding that using Information Protection labels that are linked to policies which include encryption mean that they not getting the search results they are expecting.
In summary, it’s not about using LLM’s like ChatGTP or GTP4 instead of a search engine, its about using the natural language capabilities of Generative AI tools based on Large Language Models, to automatically generate lots of metadata, and to provide conversational UI’s to enhance the overall user experience for enterprise search.
This article was originally published on LinkedIn. It has been edited and republished with the permission of the author.
About Jed Cawthorne, MBA, CIP, IG
Jed is an experienced strategy consultant, Information & Knowledge Management (IKM) practitioner and and enterprise search expert. He is an Association of Intelligent Information Management (AIIM) member of over 20 years, a Certified Information Professional (CIP), and an AIIM award winner for my work in organizing and advertising the Toronto (First Canadian) chapters activities, and a member of the 2022 intake to the AIIM Company of Fellows.