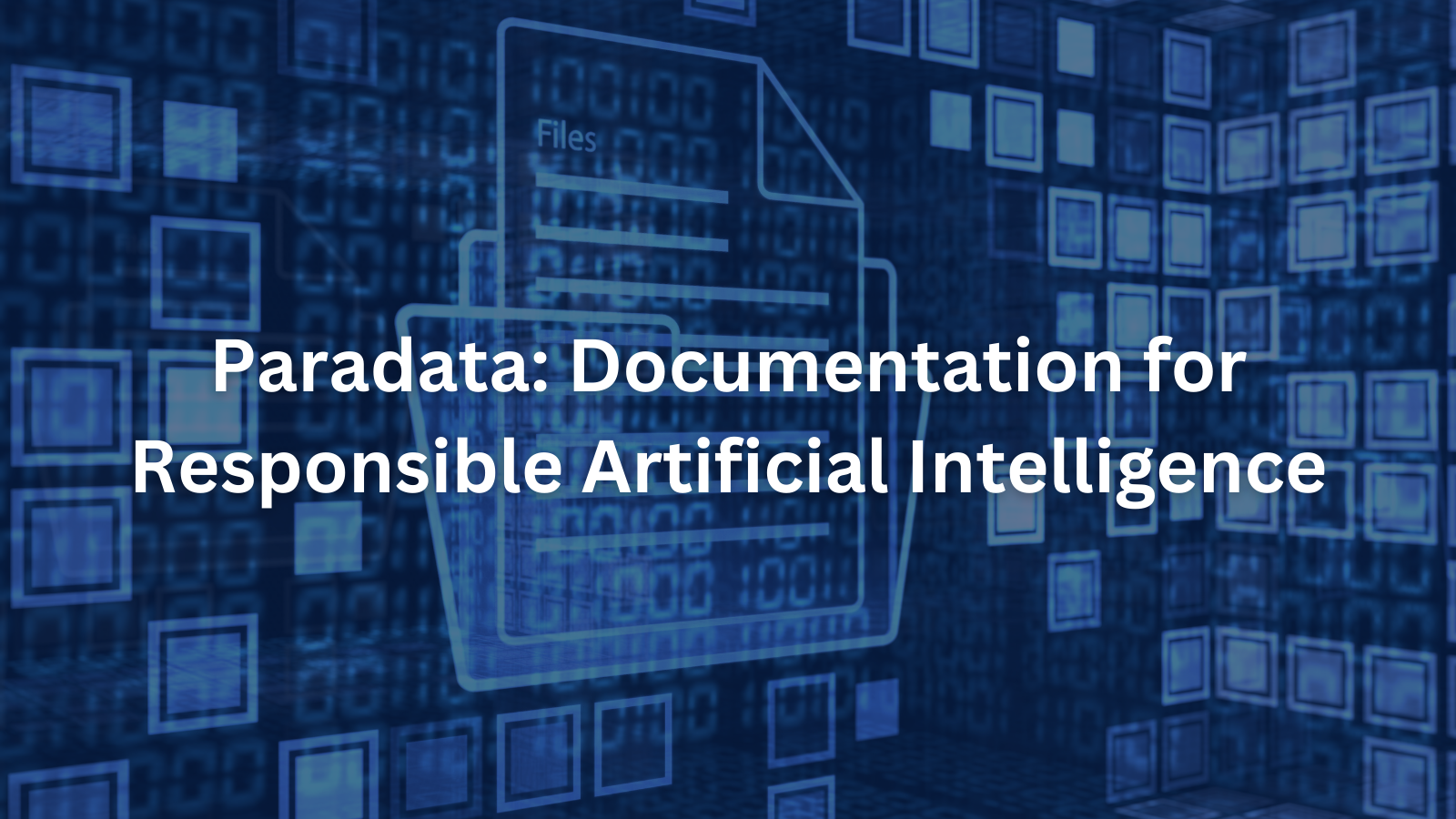
Paradata: Documentation for Responsible Artificial Intelligence
Metadata | Artificial Intelligence (AI)
Learn about paradata and how it can be used for documenting AI processes. This special guest blog post was authored by:
- Patricia C. Franks, School of Information, San Jose State University, California, USA
- Scott Cameron, School of Information, University of British Columbia; Vancouver, Canada
Importance of Accountability
While offering obvious efficiencies, the implementation of Artificial Intelligence (AI) systems raises a new set of risks including loss of trust, data breach, discrimination, and resistance to oversight.
Organizations employing AI remain accountable to their stakeholders (i.e., citizens, employees, shareholders, customers, and society) for any harm they may cause. Current research projects to make AI explainable (XAI) to human users address the technical challenges of developing human-comprehensible AI tools. But accountability within an organization requires a record of what was done by the technology and evidence that the organization has acted in a legally defensible manner.
Accountability within an organization requires a record of what was done by the technology and evidence that the organization has acted in a legally defensible manner.
Information Professionals
Information professionals, acting as stewards of information within an organization, bear responsibility for documenting the AI process.
Documentation of AI algorithms, data sources, and decision-making processes can help users, stakeholders, and regulators understand and trust AI systems, fostering responsible AI adoption. For this reason, the InterPARES TrustAI research team recommends the preservation of paradata to document the AI process. Paradata provides a framework for understanding the responsibilities of information professionals in relation to specific technical and organizational needs when applying AI tools.
Figure 1: AI Lifecycle. Source: AI Guide for Government: A Living and Evolving Guide to the Application of Artificial Intelligence for the U.S. Federal Government, GSA, Centers of Excellence. https://coe.gsa.gov/coe/ai-guide-for-government/understanding-managing-ai-lifecycle/index.html
Introducing Accountability into the AI Process
The lifecycle of an AI tool, depicted in Figure 1, moves from design through development and finally to implementation in the deployment phase.
AI tools frequently operate as black boxes impossible for humans to understand, as the complex interrelations between sophisticated models and vast training datasets are difficult to predict or reconstruct.
While the AI lifecycle is well-understood, the problem of incorporating accountability, understandability, and transparency into the process challenges researchers and legislators worldwide. The UK’s Royal Society, for instance, proposes that explainable AI is characterized by interpretability, explainability, transparency, justifiability, and contestability.
While interpretability, explainability, and transparency pertain mostly to datasets and algorithms, attention must also be paid to justifiability and contestability. How can AI outcomes be documented in a way the end user can understand? And if the users or subjects disagree with the outcome and pursue redress, what information will be available to them?
What is paradata?
Given the risks introduced by AI, organizations need to document both the actions in which AI tools participate and the processes of implementing and operating AI. To facilitate the achievement of explainability, justifiability, and contestability in AI applications, InterPARES recommends capturing paradata as “information about the procedure(s) and tools used to create and process information resources, along with information about the persons carrying out those procedures.”
In AI applications, paradata documents the organizational and technical context for AI decision-making in applications where accountability is necessary. Paradata allows for the reconstruction of the AI process, documenting due diligence in implementation to mitigate the risk of liability. The extent of the documentation required will depend on the level of risk the organization assumes and the degree of harm inherent to the application’s context.
Paradata and AI Risk Management
In practice and increasingly in response to legislation, those implementing AI tools rely upon risk management frameworks to assess the potential harms of an AI application. Higher-risk applications should retain thorough paradata as a record of their process from design through deployment and use. The European Union’s proposed Regulatory Framework on Artificial Intelligence provides a layered risk-based approach to AI applications for AI developers, deployers, and users (Figure 2). It segments AI use into four categories of risk: unacceptable, high, limited, and minimal.
Figure 2. Four levels of AI risk, EU’s s Regulatory framework proposal on artificial intelligence, last update 29 September 2022, https://digital-strategy.ec.europa.eu/en/policies/regulatory-framework-ai
The four categories help information professionals understand the extent of paradata necessary for a given application. AI applications in the unacceptable risk category will be prohibited and will not pose documentation problems for organizations. Minimal-risk applications will not require substantial documentation of the AI process. However, high- and limited-risk applications pose greater risks and require greater process documentation to demonstrate good faith and due diligence by those implementing them. This is where documentation in the form of paradata is essential.
In high-risk applications, detailed paradata may provide all information necessary about the system, its purpose, and its operations, both for authorities to assess compliance and for users to understand decisions that may affect them. Organizations must evaluate and mitigate the risk of harm in the AI systems they implement and document this process. This paradata must address each application based on its unique context.
As AI technology develops, more complex AI models will emerge. Some high-risk applications, such as those in education or employment, may continue to use understandable rule-based or decision-tree models. But more advanced and opaque machine learning or neural network tools will be developed and deployed in high-risk situations that will be more difficult if not impossible to fully understand. Processual documentation in the form of paradata will become even more important. For instance, in 2014 Amazon used a machine learning hiring tool trained on its current staff’s resumes to select applicants to advance in their hiring process. However, Amazon scrapped the tool in 2018 upon realizing the tool reproduced existing hiring biases favoring male applicants.3 In similar cases, documentation requirements for the implementation and operation of AI tools may reduce risk by obligating implementers to complete their due diligence before operationalizing a tool.
Types of Paradata
Paradata documents both the technical aspects of a given tool and the organizational process of its implementation. Technical paradata documents the nature and operations of a model. This may include the model’s training dataset, versioning information, evaluation and performance metrics, logs generated, and existing documentation provided by a vendor. Organizational paradata documents the social and organizational context of the tool’s use. This may include documents speaking to design, procurement, or implementation processes, relevant AI policy, and ethical reviews conducted.
Taken together, this cohesive package of paradata may be used to document and explain AI applications employed by an individual or organization. Approaching the challenge of documenting the AI process, information professionals who may not be embedded in the AI implementation process from the beginning will need to take an active role to ensure the creation and preservation of the paradata necessary to document the AI process.
Conclusion
Much work remains in understanding how to document the AI process for risk management and accountability. In elevated-risk applications, organizations and individuals using AI must consider how to make their AI implementations transparent to their stakeholders both in the present and into the future. What is certain is that the creation and preservation of paradata has the potential to foster ethical AI development and deployment, while addressing concerns related to bias, privacy, algorithmic accountability, and transparency.
About Patricia C. Franks
Dr. Patricia C. Franks is a Certified Archivist, Certified Records Manager, and Information Governance Professional, as well as a member of ARMA International’s Company of Fellows. She is co-editor of the Encyclopedia of Archival Science, the Encyclopedia of Archival Writers, 1515-2015, and the International Directory of National Archives. She is author of Records and Information Management now in its second edition. She was team lead on the 3DPDF Consortium’s 2020 Whitepaper, Understanding Blockchain’s Role and Risk in Trusted Systems.