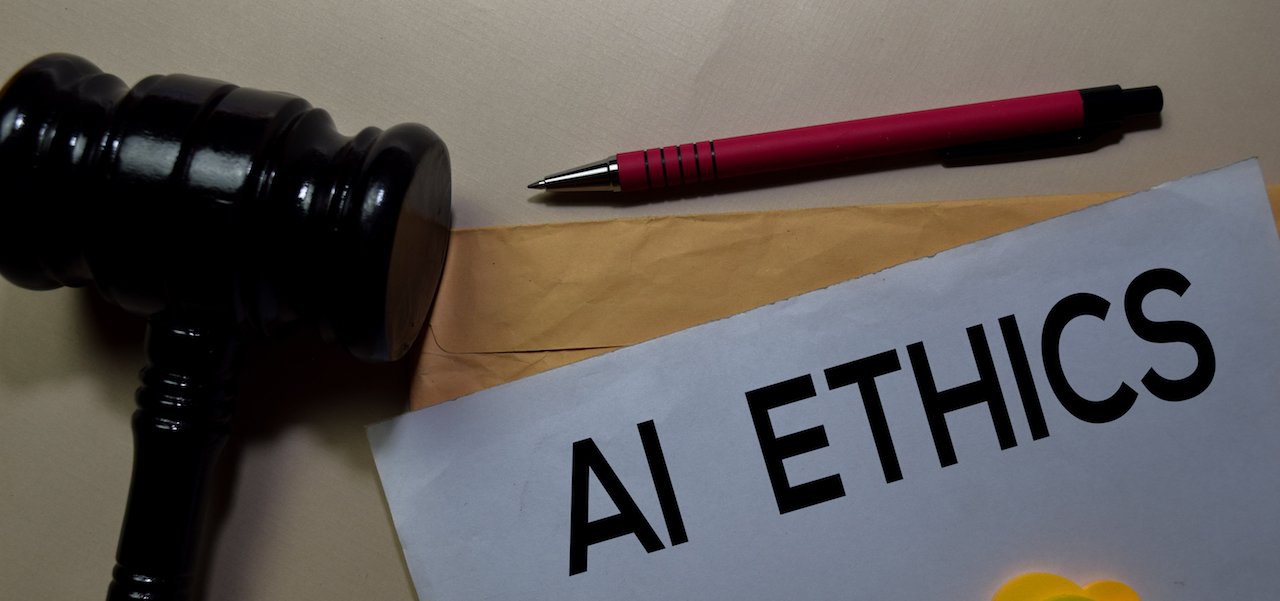
Ethical Use of Data for Training Machine Learning Technology - Part 1
Machine Learning | Artificial Intelligence (AI)
This is the third part of a 3-part series on the Ethical Use of Data for Training Machine Learning Technology by guest authors Andrew Pery and Michael Simon. You can also check out Part 1 and Part 2 from this series.
Part 1: Bad Things Can Come from Non-neutral Technology
AI technology is becoming pervasive, impacting virtually every facet of our lives. A recent Deloitte report estimates that shipments of devices with embedded AI will increase from 79 million in 2018 to 1.2 billion by 2022: "Increasingly, machines will learn from experiences, adapt to changing situations, and predict outcomes…Some will infer users' needs and desires and even collaborate with other devices by exchanging information, distributing tasks, and coordinating their actions."
The advances in and social utility of AI technology notwithstanding there are serious concerns relating to unintended consequences of AI technologies as “such systems are replicating patterns of bias in ways that can deepen and justify historical inequality.”
A symposium facilitated by AI Now Institute framed the challenges associated with AI as follows:
“The problems raised by AI are social, cultural, and political — rather than merely technical. And despite the rapidly increasing use of AI in many spheres, it’s imperative to step back and decide just what types of AI are acceptable to us as citizens and how much we should actually be relying on technologies whose social costs have not yet been fully discerned.”
3 Examples of Bad, Biased, or Unethical AI
1. Facial Recognition
None more consequential is the use of facial recognition technology. For example, The US Government is deploying a pilot facial recognition system at the Southern Border that records images of people inside vehicles entering and leaving the country with the "ability to capture a quality facial image for each occupant position in the vehicle." Evidently, the US government secretly collected large volumes of data in Texas and Arizona of people presumably from immigrant populations doing their daily chores, going to work, and picking up their children from school. A similar recent report of troubling use of this technology was documented by Georgetown Law's Center on Privacy and Technology study, and reported in major national news media, as to how US Immigration and Customs Enforcement (commonly known as "ICE") analyzed millions of driver's license photos in three states without their knowledge. As was observed by the Executive Director of Media Justice: "This is an example of the growing trend of authoritarian use of technology to track and stalk immigrant communities."
Such examples of abuses of civil rights are rampant. Moreover, such systems have been proven to be wildly inaccurate, as recent studies in the UK show, up to 96 percent. Just recently, as part of a continuing study, the US National Institute of Standards and Technology ("NIST") tested 189 software algorithms from 99 developers that were used for common facial recognition scenarios. NIST's report found "a wide range inaccuracy across developers," and identified two disturbing issues:
- Higher rates of false positives in US-developed systems for Asian, African American, and "native group" demographics (especially American Indian") faces for one-to-one matching (used for ID verification purposes) of 10 to 100 times.
- Higher rates of false positives in a number of systems for African American females for one-to-many matching (used for identifying potential suspects).
Even some of the innovators of facial recognition technologies, such as Microsoft, have recognized the need to resolve bias issues, per the admissions of its President, Brad Smith: "The technologies worked more accurately for white men than for white women and were more accurate in identifying persons with lighter complexions than people of color." Indeed, studies by MIT researcher Joy Buolamwini found that Microsoft's facial recognition software had an error rate of 1% for white men and more than 20% for black women. An ACLU study found that Amazon's "Rekognition" software identified 28 black members of Congress as criminals. Some of these concerns have led to the recent decision by cities such as San Francisco, Oakland, and two suburbs of Boston to ban the use of such technologies. Unfortunately, as this very recent article in Politico details, efforts to regulate facial recognition on a national level, both in the US and the EU, have been effectively stalled by a variety of setbacks.
2. Criminal Justice
A report published by Pro Publica found that COMPAS, the most popular software used by US courts for criminal risk assessment mistakenly labeled visible minority offenders as more likely to re-offend at twice the rate as white offenders. COMPAS also mistakenly labeled white offenders as twice as likely not to re-offend than non-white offenders. The then-Attorney General Eric Holder has expressed concerns about the potential inequities raised by the application of AI technology to model and predict recidivism rates within the offender population:
"Although these measures were crafted with the best of intentions, I am concerned that they inadvertently undermine our efforts to ensure individualized and equal justice. They may exacerbate unwarranted and unjust disparities that are already far too common in our criminal justice system and in our society."
Unsurprisingly, "Predictive Policing" systems designed to detect potential patterns in criminal activity to allow police to prioritize resources or even intervene in time, have come under similar concerns about bias and lack of transparency.
One would think that legal experts would want to take a careful look behind the "curtain" of systems that can help put people in jail and keep them there longer. While the company that created COMPAS has claimed that race is not a factor in the COMPAS algorithm, the company has refused to provide full access to its algorithms. Unfortunately, the Wisconsin Supreme Court explicitly declined such an opportunity in Wisconsin v. Loomis, 2015AP157-CR, (July 13, 2016). Instead of critically examining the suspect system, which the criminal defendant, in that case, claimed gave him a higher recidivism score than warranted, the court simply accepted the accuracy of the system and merely gave trial court judges a weak caution against relying on it too much. Even worse, a follow-up report by two data scientists from Dartmouth last year found that a random group of inexperienced crowd-sourced individuals performed better using just 2 factors for testing than the 137 factors of the COMPAS system.
Fortunately, there has been some movement lately on requiring better accountability in algorithms used in criminal matters. Congressman Mark Takano (D-CA), introduced the "Justice in Forensic Algorithms Act of 2019" in September 2019 to require federal standards and testing of all such systems before use. As Rep. Takano told news-site Axios: "We need to give defendants the rights to get the source code and [not] allow intellectual property rights to be able to trump due process rights."
At the beginning of last July, Idaho became the first state to pass a law specifically requiring transparency and accountability in pretrial risk assessment systems. As well, last summer, the San Francisco District Attorney, in conjunction with the Stanford Computational Policy Lab, implemented an open-source bias mitigation tool to assess prosecutors' charging decisions. Others at Stanford, including professors in the university's Human Rights Data Analysis Group, have been working to develop ways to "repair" potentially biased data in the criminal context.
3. Benefits Entitlement
Increasingly, AI-based applications are used to adjudicate entitlement benefits such as eligibility for unemployment insurance. For example, the State of Michigan implemented the Michigan Integrated Data Automation System. This “robo-adjudication program falsely accused tens of thousands of unemployment claimants of fraud.” The unintended, yet profound impact were “severe, seizure of tax refunds, garnishment of wages, and imposition of civil penalties – four times the amount people were accused of owing.”
A subsequent state review of the inherent flaws of the application found “that from October 2013 to August 2015 the system wrongly accused at least 20,000 claimants of fraud, a staggeringly high error rate of 93 percent.”
The State of Michigan is now subject to a pending class-action suit claiming that: “This system and software, including its design and implementation, is constitutionally deficient and routinely deprives individual unemployment claimants, who are some of the state‘s most economically vulnerable citizens, of their most basic constitutional rights.”
What Can We Do About Biased AI?
By now, it should be clear that significant challenges remain regarding the use of AI, especially with respect to the potential for bias in decision making. These challenges may be the results of poorly-designed algorithms, algorithms based on invalid assumptions, or biased data sets, but all of them can cause immediate and sometimes irreparable harm to individuals and organizations alike. So long as we believe that software cannot be biased, these types of issues will only increase.
In the next part of this series, we'll offer suggestions for how to make AI more ethical - or at least lead to more ethical outcomes.
About Andrew Pery
Andrew Pery is a marketing executive with over 25 years of experience in the high technology sector focusing on content management and business process automation. Currenly Andrew is CMO of Top Image Systems. Andrew holds a Masters of Law degree with Distinction from Northwestern University is a Certified Information Privacy Professional (CIPP/C) and a Certified Information Professional (CIP/AIIM).