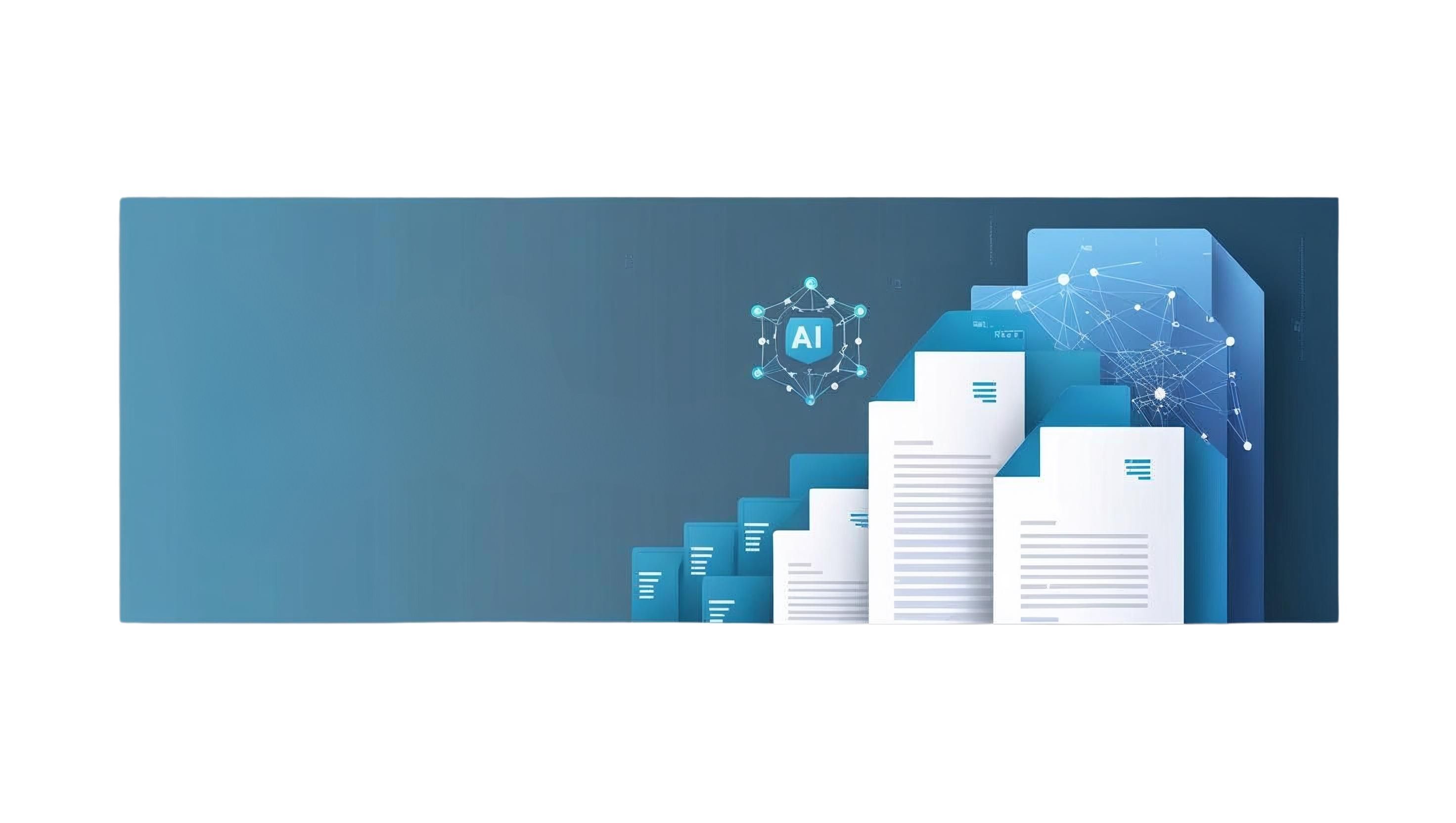
Why We Can’t Use Machine Learning to Automatically Classify All Records
Electronic Records Management (ERM) | Machine Learning | Artificial Intelligence (AI)
It sometimes feels like the battle for control of unstructured data is being won by the forces of employee document hoarding and data over-retention. The unchecked growth of files, emails, and other content drives up online storage costs, undermines privacy-driven data minimization efforts, increases eDiscovery risks and expenses and, perhaps most importantly, chokes employee productivity and collaboration.
The result? Too much digital clutter. “Tell me something I don’t know,” says every information governance professional.
In response, some organizations are turning to artificial intelligence, specifically machine learning (ML), to classify, manage, and especially delete expired records and low-value information.
ML, a type of AI that has been around for years, powers tools like Microsoft 365 Purview’s trainable classifiers. Other platforms, such as BigID and OneTrust, also promote ML-driven classification. These systems are great at pattern recognition (for example, identifying elements like credit card numbers or Social Security numbers) which makes them quite effective at detecting certain types of personal information or other sensitive data. With capabilities like these, it is easy to believe ML might finally be the answer to the problem of records sprawl.
Why Machine Learning Falls Short
But while machine learning can successfully identify and classify certain types of information, it is not a viable strategy for classifying most records.
Vendors offer a mix of "out-of-the-box" classifiers for commonly recognized data types along with custom, trainable classifiers that users can build themselves. The assumption is that the out-of-the-box classifiers will catch the majority of records and the custom ones can round out the rest.
In practice, though, many records don’t contain the kinds of obvious keywords or consistent formats that ML models rely on. As a result, they often defy automated classification. And even well-trained models can make critical mistakes. For example, if a system misclassifies an approval document as trivial, it might be deleted. Conversely, multiple drafts or convenience copies of a low-value document could be retained unnecessarily, adding to the clutter.
Some vendors claim that layering custom classifiers on top of standard ones will get classification coverage to acceptable levels. In reality, you might get 40% of the way there with out-of-the-box models and another 10% of the way with initial custom classifiers, but diminishing returns from there on out. The next round of classifiers may only capture an additional 5%. The initial results feel successful. But like a tractor pull at a county fair, the first few yards are easy while every yard after gets exponentially harder.
There are tools that boast the ability to create 3,000 or more custom classifiers. However, building, testing, and maintaining so many classifiers is a herculean task. For most organizations, this approach is not scalable or sustainable. Telling a regulator that we have successfully classified 65% of our records simply doesn’t cut it. In short, while ML is helpful for some types of data classification, it is not a feasible core strategy for enterprise-wide records management.
A Better Approach: Data Placement and Automation
So should we give up on solving over-retention? Absolutely not.
There’s another strategy that does work: data placement and automation. Rather than asking AI to figure out what something is, this approach trains the content management system to apply the right rules when content is stored in the right place.
With tools like SharePoint, Teams, and OneDrive, organizations can configure managed folders with built-in metadata inheritance. When employees drag and drop files or emails into these folders, the system automatically applies the appropriate retention and sensitivity labels. Management, access control, and disposition are fully automated from that point on.
While this is not “true” auto-classification (since it requires users to spend a few seconds choosing the right folder), it’s close. Once the content is placed, the rest of the governance process is fully automated.
Even better, this approach doesn’t require purchasing expensive new products. If your organization uses Microsoft 365, the standard E3 license includes all the necessary retention labeling functionality. Other than a few specialized users in eDiscovery, InfoSec, or records management who may benefit from E5 features, no enterprise-wide upgrade is required. Yes, there’s some up-front work to configure managed areas and train users, but once deployed, this model scales effectively even in large, complex, global environments. Employees find it intuitive, and organizations gain consistent, policy-based retention and defensible compliance.
ML Still Has a Role, Just Not the Lead
This isn’t to say ML has no value in information governance. It can be highly effective for identifying sensitive data, supporting ROT remediation, and surfacing security risks. But it’s not the “be-all and end-all” of records classification. We must match the right tools to the right problems. When it comes to large-scale records classification, sorry, but ML alone can’t get us there.
Looking Ahead: Generative AI Will Do What ML Cannot
Eventually, generative AI may succeed where ML has struggled. One day we may trust AI to classify records with accuracy that meets or exceeds human judgment. But for now, applying generative AI at enterprise scale is still too computationally expensive for routine use in classification and governance.
Until then, the smartest strategy blends data placement and automation with selective, targeted use of ML. This reduces the chaos today while laying the foundation for organizations to benefit from smarter automation tomorrow.
About Mark Diamond
Mark Diamond is the founder and CEO of Contoural, the largest independent provider of information governance, privacy, and AI governance strategic consulting services. He and his firm work with more than 30% of the Fortune 500 in addition to many mid-sized companies, public sector, and nonprofit organizations. As an independent provider, Contoural neither sells products nor takes any referral fees. Mark welcomes discussion and debate on this and other topics. Email him at markdiamond@contoural.com.